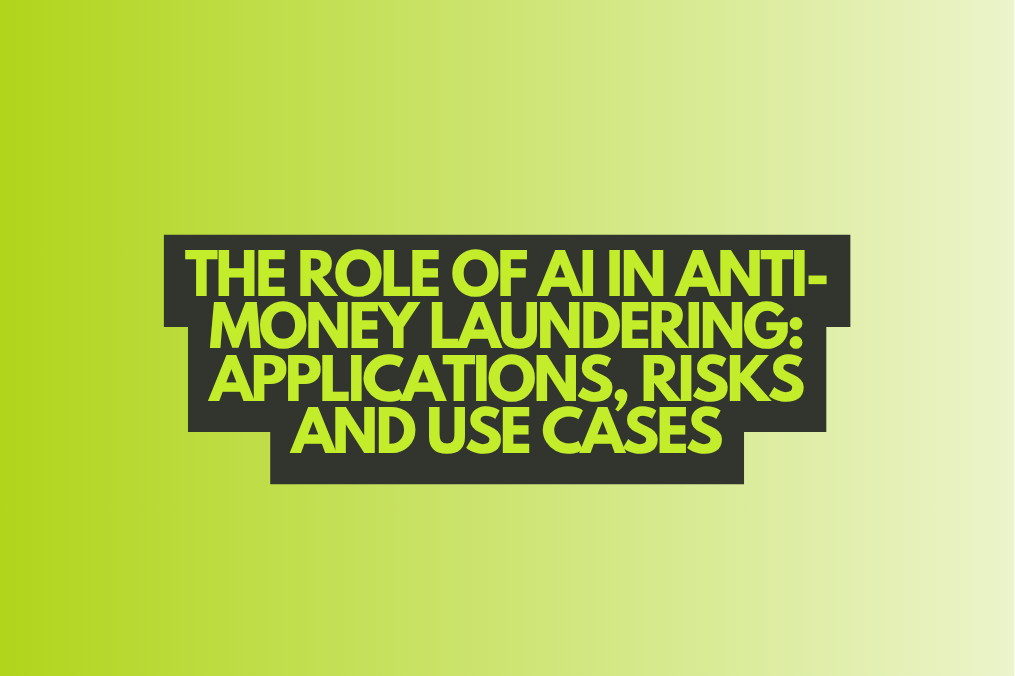
The Role of AI in Anti-Money Laundering: Applications, Risks and Use Cases
Anti-Money Laundering (AML) is getting a boost from Artificial Intelligence (AI). This article explores how AI is revolutionizing AML by detecting hidden criminal patterns, but also discusses the challenges of data privacy and ensuring fair AI models.
The intersection of Artificial Intelligence (AI) and Anti-Money Laundering (AML) is a fascinating area of study. It's a dynamic field where technology meets regulatory compliance, creating a powerful tool in the fight against financial crime.
AI's ability to analyse vast amounts of data and identify suspicious activity is revolutionising AML processes. However, understanding this connection is crucial to fully harness its potential and navigate the complexities of regulatory standards. In this blog post, we'll delve deeper into this intriguing relationship.
The Role of AI in AML Compliance
AI's role in AML compliance is becoming increasingly significant. It's not just about automating tasks, but also about enhancing the effectiveness of AML efforts. AI models can continuously learn and adapt to new patterns and trends, helping to identify previously unknown risks. This improves the overall accuracy of AML programs, making them more robust against financial crimes.
AI systems can help financial institutions navigate the regulatory complexity associated with AML. They can flag suspicious activities, ensuring compliance with international standards. This not only reduces the burden on these institutions but also aids law enforcement agencies in their fight against financial crime.
{{snippets-guide}}
Anomaly Detection and AI
AI technology has revolutionised the financial system, particularly in the realm of Anti-Money Laundering (AML). One of the key applications is anomaly detection. This AI technique is used to identify unusual patterns or behaviours that deviate from the norm.
In the context of AML, anomaly detection helps banks to spot suspicious transactions that could indicate money laundering. This use case for AI not only enhances fraud detection but also aids in risk assessment, making it a vital tool in the fight against financial crime.
Pattern Recognition and AI
Pattern recognition is a crucial application of AI in AML. AI models, trained with labelled data, are adept at detecting specific criminal patterns that might otherwise go unnoticed with traditional methods. This use case for AI is particularly effective in automating the identification of complex patterns, enabling rapid and large-scale detection.
One such AI technology that enhances pattern recognition is Large Transaction Models (LTMs), pioneered by Hawk AI. LTMs excel at connecting behaviour across massive datasets, identifying patterns across long "distances" within the data. This ability to find the 'needle in the haystack' makes LTMs a powerful tool in the fight against money laundering.
False Positive Reduction and AI
AI false positive reduction technology is a game-changer in the realm of Anti-Money Laundering (AML). This AI technique is designed to refine the detection process, minimising the number of incorrectly flagged alerts that AML teams need to review. By creating models of expected transaction behaviour, it provides more precise definitions of normal and abnormal activity, thus reducing the burden on compliance teams.
The technology also ensures compliance with new regulations by providing audit trails for every alert. This feature promotes the risk-based approach required by regulators, making it an invaluable tool in the financial system. The use case for AI in this context is clear - it enhances the efficiency and effectiveness of AML teams, helping them achieve their organisational goals.
pKYC and AI
AI technology is playing a pivotal role in the implementation of pKYC, a crucial component in mitigating AML risk. This technology utilises dynamic factors derived from AI models, such as suspicious behaviour, to generate accurate and current risk assessments. Without the detailed customer risk view that AI provides, pKYC would be virtually unachievable.
The risk profiles of customers can fluctuate rapidly. AI-powered pKYC enables AML teams to monitor customer behaviour for these changes effectively, ensuring a balanced risk portfolio. This allows banks to adopt a risk-based approach to AML compliance, safeguarding their reputation and preventing financial crime. With potential new regulations requiring pKYC processes, the importance of AI technology is set to increase.
Data Privacy Concerns in AI AML
The application of AI in Anti-Money Laundering (AML) has raised significant data privacy concerns. As AI models are trained on vast amounts of customer data, there's a risk of sensitive information being misused or falling into the wrong hands. This is a major concern for banks and financial institutions, who are bound by strict international compliance association rules to protect customer data.
Moreover, the generative AI model used in AML can sometimes make a decision or prediction that is not entirely transparent. This lack of transparency can lead to regulatory complexity that institutions must navigate carefully. It's crucial for these institutions to strike a balance between leveraging AI for AML and ensuring data privacy.
Risk Assessment in AI AML
The use of AI in AML is not without its risks. One of the key challenges lies in the risk and regulatory complexity that institutions face when implementing AI solutions. AI models, particularly generative ones, can sometimes produce inaccurate or misleading results, which can lead to serious legal and regulatory repercussions.
Moreover, the risk profile of a customer can be misinterpreted by AI, leading to false positives or negatives in detecting suspicious behaviour. This could potentially result in referral fraud, causing significant damage to both the bank and financial institution's reputation and bottom line. Therefore, a thorough risk assessment is crucial when integrating AI into AML processes.
{{snippets-case}}
The Future of AI in AML Compliance
The power of AI to detect suspicious activity, ensure compliance, and reduce false positives is transforming the way financial institutions tackle financial crime.
As AI models continue to evolve, they will become even more effective at identifying suspicious behaviour and assessing customer risk. This will not only help banks and other financial institutions to minimise referral fraud, but also to meet new regulations and standards set by international compliance associations.
At sanctions.io, we've been using AI technology to assist our clients and improve the accuracy of the screening process. If you are a a major financial institution, fintech company, or any oher institution concerned about compliance, AI can be a life-saver. To learn more about how our AI-driven sanctions, PEP, and criminal watchlist screening service can support your organisation's compliance program, book a free Discovery Call.